The program was organized for (aspiring) Data Science students, graduates, people in their early careers and more seasoned professionals that consider switching careers from another field. 300 attendees from 15+ different countries watched us live on Zoom where we covered topics such as:
Day 1 (for summary & highlist go here)
- Why choose a Data Science career?
- Data Science job & career perspectives
- How to choose a program and institute?
- Understanding titles: ‘Who is who in the Data Science team’
- Skills: ‘What languages and tools to learn’
- What’s it like working as a Data Scientists (corporate vs entrepreneur)
Day 2
- Leadership skills for Data Scientists
- What’s it like working at a Machine Learning consultancy firm
- What recruiters look for in a resume
- How to prepare for your job interview
- Data Science case presentation
Here is a link to the full program and line-up: https://techminds.elu.nl/data-science-career
Meeting the Hosts
AIgents (aigents.co)
AIgents is a career community for Data Science and Machine Learning Engineers. It's used to discover career opportunities (like jobs and internships), events and training courses. You can join their Machine Learning Meetup or one of their LInkedIn groups to keep track of developments in your field of study. To connect, learn and collaborate with other students and professional go to 'Masters in AI' learning community.
Founded by Robbert Van Vlijmen, the community has become one of the largest tech communities in Europe.
European Leadership University (elu.nl)
The European Leadership University is a next-generation university focused on filling the skills gap that businesses currently face. Founded by Alper Utku, the university consists of programs based on the job market, investing in young professionals to give them the skills they need now.
Leadership Skills for Data Science — Mini-Workshop
This is a summary of a workshop by Alper Utku - President at the European Leadership University - given at the Data Science Career Summit 2020. You can find a full video of this workshop here.
Table of contents
- Why do we need leadership skills in data science?
- Self Leadership
- Team Leadership
- Organizational Leadership
- Community Leadership
- How can we start applying these skills?
Why do we need leadership skills in data science?
We can look at leadership not only from a power and authority perspective but also from a relational perspective: a way of trying to improve ourselves in different levels of relationships. In data science, we need leadership skills to effectively communicate with colleagues and stakeholders, to solve problems, collaborate and to create connections with people in the field.
As a data professional, you will be engaging at different levels. We start with self-relationship awareness which will also be used at other levels such as one-to-one leadership, team leadership. Then you can go beyond the teams that you operate in, which is what we call organizational skills.
Self Leadership
It's recommended that - as a data scientist you have a coaching mindset and develop skills to use at all levels of your one-to-one relationships. A few skills that are crucial at this level include:
- Reflection
- Patience
- Discipline
- Empathy
- Pragmatism
- Interpersonal communication
Team Leadership
At the team level, we take our coaching mindset to group levels. When we engage with teams, we need an approach that gives us a little bit of structure but also enough room for creativity.
If you want to do well in data science and work with groups, either in a virtual or physical way, it's strongly recommended that you work on your facilitation skills. Thus, you have to bring the voice of your team, get their input and be able to produce something together. A few skills involved at this level are:
- Empathy
- Trust
- Communication
- Negotiation
- Patience
- Listening
Organizational Leadership
Organizational leadership involves the skills we need to engage with the greater organization, with a company. At this level, you will be motivating, influencing and getting people on the same page. Therefore, you have to be a trusted advisor with consultancy skills.
Whether you are an internal or external consultant, you need to have a trusted advisor mindset. Skills involved are:
- Understanding the needs of the organization
- Being the connection between different parts and departments
- Understanding the business acumen
- Domain expertise
- Strategic vision
Community Leadership
At the community level, you want to take your word out and contribute to the data science community. Here, we can include any practices of writing, producing and sharing knowledge. This type of leadership is very important to succeed in the field. We can add skills such as:
- Mentoring young professionals
- Great writing and verbal skills
- Blog writing
- Producing videos
How can we start applying these skills?
The best starting point is working on your growth mindset and taking everything as a learning opportunity. Then, you will take this growth mindset to build up new skills. When you start by developing a coaching mindset, you can expand this to broader settings like teams and organizations, and even communities.
A Day in the Life of a Data Science Student - Interview
This is a summary of an interview with McAndrew Saad - Data Science Master's Student - given at the Data Science Career Summit 2020. You can find a full video of this interview here.
McAndrew Saad has a degree in Computer Science and works as a software consultant. Currently, he is a master's student in the ELU (European Leadership University) Data Science programme. He answered questions about his experience with the program so far.
How was your learning experience in the data science master's programme?
— I like the program because it doesn't follow traditional university techniques. Instead, the program is a lot more practical, including courses and projects. Most of the work has to be done by yourself, which teaches you a lot about being responsible and have initiative. Besides, the program is offered online and it's part-time, so I can have a work-life while also staying up-to-date on my studies.
How do you feel that getting a master's will prepare you for your future career?
— Education alone doesn't have what it takes to become a successful data scientist. This masters will serve as a solid foundation from which I will build on with extra courses and projects that would complement my studies and give me some practical experience. You need a lot of practical work experience to become a really good data scientist.
What are some skills you have picked up since you started studying data science?
— From a leadership and team perspective, I learned a lot about collaboration, communication and coaching. How to work within a team and how to organize yourself. One of the experiences was owrking on group projects where I had to work with people from different countries and continents. At the technical level, everything I know now about Data Science I acquired through this 10 months program.
What are your career plans?
— My future plan is to find a job in Europe or Canada, where we have big data science centers with a lot of data science jobs available. The more I dive into data science, the more I find that this field will keep growing because you can make data (science) out of everything, even in small businesses.
What has surprised you since you started to learn and practice data science?
— To be successful you have to do a lot on your own, you have to be an initiator. Research, find new tools, trends and learn by yourself and by doing. You must be able to learn new things and improve your skillset all the time.
What piece of advice would you give to someone who thinks about starting studying data science?
— I would say to know precisely what you want. Many people confuse the different parts of data science, so it's very important to do research and find out exactly what you want to do in the data science field.
What Is It like Working at a Machine Learning Consultancy Firm?
This is a summary of a presentation by Robbe Sneyders - Head of Delivery at ML6 - given at the Data Science Career Summit 2020. You can find a full video of this presentation here.
Robbe is head of delivery at a machine learning consultancy firm (ML6). He has more than three years of experience as a machine learning engineer.
Table of Content
- ML6
- What is a machine learning services company?
- Projects at ML6
- Teams at ML6
- Machine Learning Engineer skills
- What is the most important skill for a Junior applying to ML6?
ML6
What is a machine learning services company?
Different from product companies, a service company doesn't build its own products but sells services to its clients to help them build their products. This has some advantages, since consultants work on a lot of different projects, they have many opportunities to take ownership, responsibility, learn a lot and grow rapidly.
Projects at ML6
There are three types of projects or stages developed at ML6 (check below).

The first one is a proof of concept, which is the first project for a new client to assess the technical feasibility of a solution and to demonstrate that intelligent technology can add value to their problem. In this phase, it's shown what can be done with their data and what kind of models could be applied.
The next step is the MVP project, where they try to put a model in an application. This is an end-to-end solution with the goal to find out if the model can perform in a real-life situation.
The last part is a project putting machine learning into production. The goal here is to set up a solution that can be fully integrated with the client systems, completely automated and that retrains the model automatically.
Teams at ML6
There are four main roles at ML6:
- Machine Learning Engineers
- Software Engineers
- Data Engineers
- Project Managers
Why only Machine Learning Engineers and not Data Scientists?
The real challenge here is not building the machine learning model but building an integrated machine learning system. If you look at a machine learning systems (image below), you see that only a very small fraction consists of actual machine learning code. Therefore, developing the model is only a small part of the whole process.
The image below summarizes the different skills needed throughout the process.

Machine Learning Engineer Skills
Machine learning engineers need to combine several skills related to machine learning: data, models, software development, deployment and systems. In services companies, everyone needs to work together directly with clients, so they need good communication skills, business understanding and problem-solving skills.
What is the most important skill for a Junior applying to ML6?
ML6 want people that can find ways to solve problems themselves and work independently while having the support needed to achieve the best results. They don't have strong requirements on experience as long as the professionals can solve problems and have a strong scientific background.
Why Choose Data Science as a Career?
This is a summary of a presentation by Jason Li - Machine Learning Engineer at ML6 - given at the Data Science Career Summit 2020. You can find a full video of this presentation here.
Jason Li has a master's degree in computer science and currently works as a machine learning engineer at ML6 in Amsterdam.
Table of Content
- Why data science is charming?
- A brief history of language models
- NLP applications
- Get paid well
- AI industries and professionals
- Workflow at ML6
Why data science is charming?
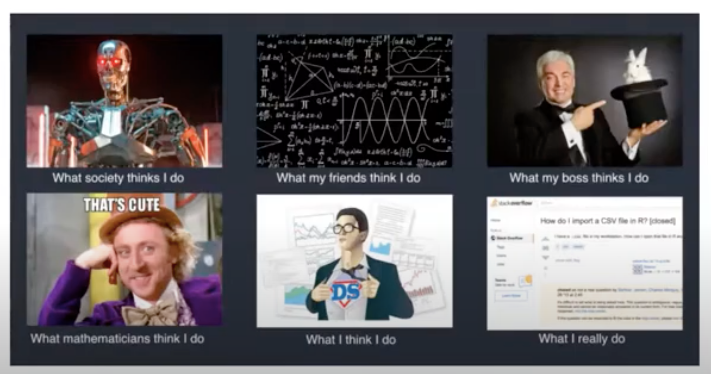
The data scientist is the sexiest job of the 21st century. That's because data scientists have the skills that are necessary for any organization wishing to profit from data.
A brief history of language models
For a long time, people want computers to be more intelligent and to be able to understand human languages for example. Their approach to achieve this was mostly based on rule-based models, where you create a program that can analyze the sentences by splitting them in pieces and trying to understand the structure using different techniques.
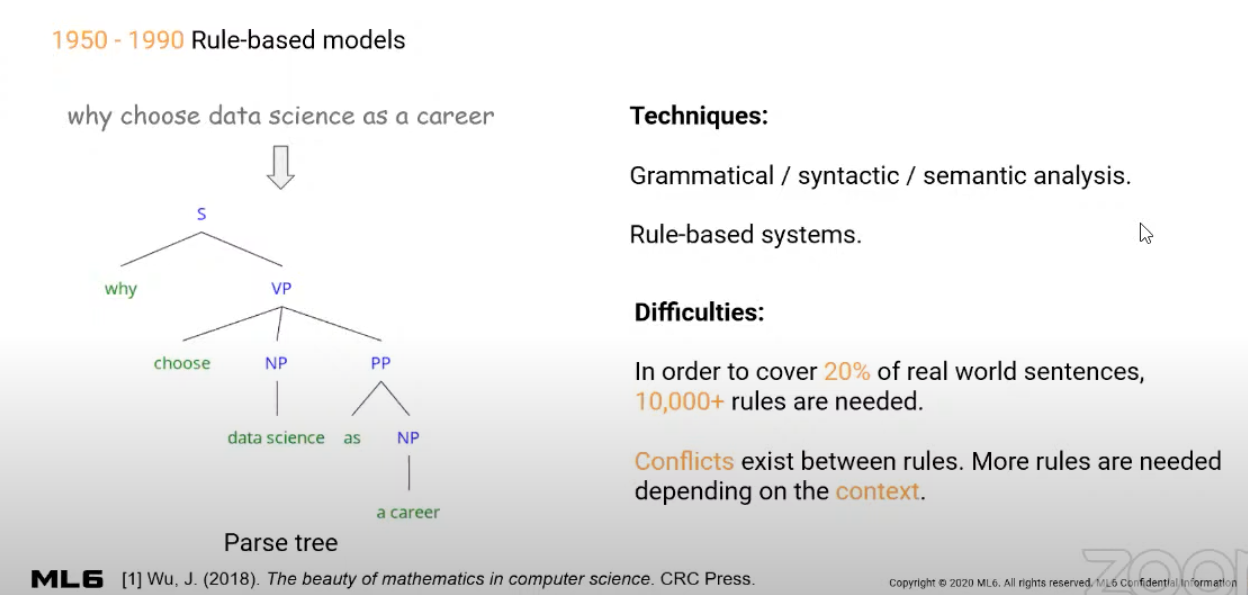
Then, they realized that they could use statistics instead of analyzing the rules of the sentences.

Because of gained computer power, deep learning was coming up as a more sophisticated approach to deal with the language problem.

NLP applications
After years of development in language models and deep learning, we have a lot of applications nowadays.

What's the difference between a Data Scientists and a traditional software developer?
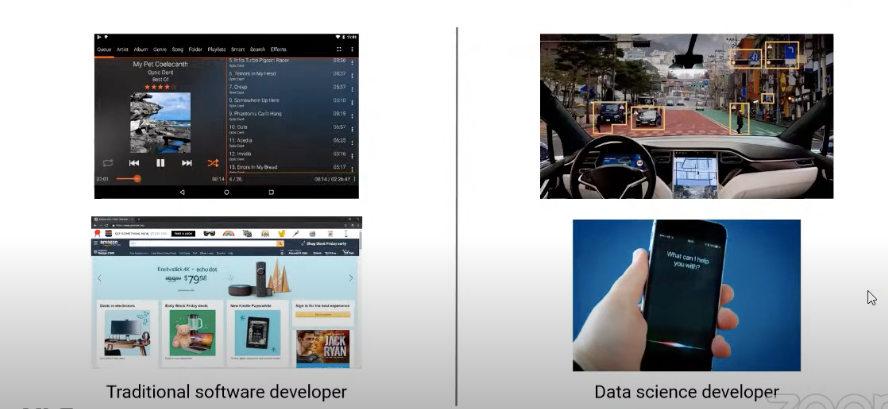
Traditional Software Developers:
As a traditional software developer, you will develop applications for web of mobile where you implement a certain logic that is predefined. Basically, they can expect certain inputs that gives back expected output.
Data Science Developer:
As a data science developer, you are trying to make computers smart to solve problems that humans can't solve. So it's more than logics. We use statistical models to do this job.
If you want to work in data science you can either be a researcher or an engineer.
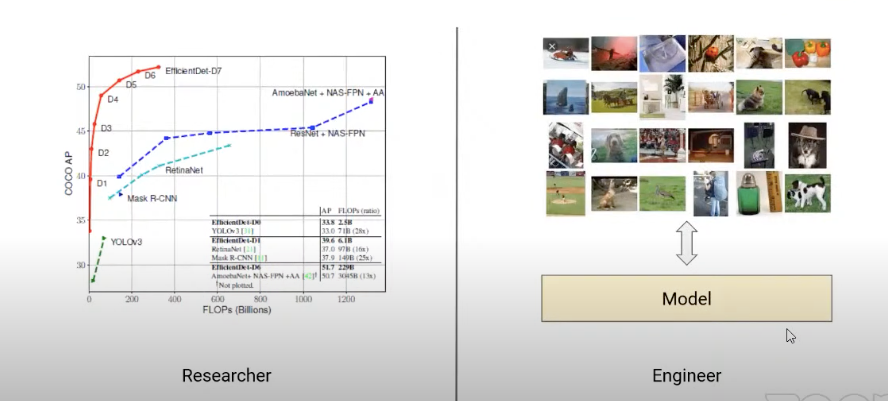
Researchers are trying to modify model architectures or push the accuracy or performance to a new level. Therefore, researchers are trying to do something that nobody has ever done. Engineers solve actual problems in the real world. Basically, they get the data and try to find the best model to fit that data and solve these problems.
Workflow at ML6
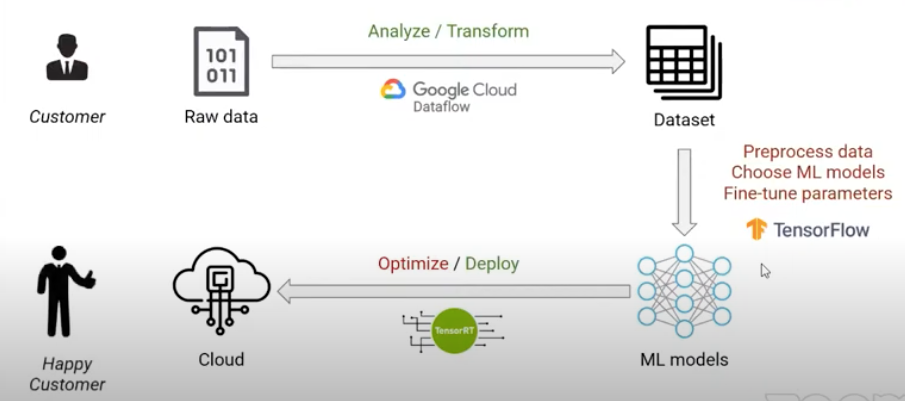
At ML6, customers provide their raw data and a problem they want to solve. The first step is to analyze and transform this data, in the case of big data they can use cloud platforms (e.g. Dataflow). Then, machine learning engineers pre-process the data, choose ML models and fine-tune the hyperparameters in order to find the best match model to fit this dataset. In the end, the model is working and they can optimize it to make it smaller and faster. Finally, the model is deployed.
Landing your Dream Job: What Recruiters Look for in a CV
This is a summary of a presentation by Casper Hoppe - owner at Hoppe Executive Search - given at the Data Science Career Summit 2020. You can find a full video of this presentation here.
Casper Hoppe runs a headhunting agency in AI (Hoppe Executive Search) and is specialized in deep learning, machine learning, computer vision and data science. He has in-depth knowledge of the IT market in The Netherlands and expanded successfully to other sectors such as AI for MedTech and customer services.
Table of Content
- How does recruitment work?
- Recruitment agency Vs Headhunter
- Working on your resume
- How to choose a domain or an industry?
- Overcoming a few challenges
How does recruitment work?
There are quite a few players in the recruitment sector. We have the parties involved within the company, like an internal recruiter and the hiring manager. There are usually also external parties included in supplying and completing job offers.

The headhunter is an external role that works based on a request from the hiring manager. Headhunters are specialized in a certain field and they work together with the hiring manager. Therefore, they have direct access to the source of information on what's needed.
Recruitment agency Vs. Headhunter
Recruitment agencies get a lot of offers from a potential employer and they work more on numbers, meaning that they source more candidates to this employer. Usually, if you are an earlier professional you can have a better chance of working through recruitment agencies but if you have more experience then you would probably be better off with a headhunter that is specialized in your field.
Working on your resume
- Format
One core recommendation is to always have your resume reviewed. There can always be a mistake or an unclarity that you missed which can be a bad sign to a hiring manager. If the person has mistakes on the resume how is he/she gonna perform working with details in data science? Therefore, be meticulous in scanning and rescanning your resume.
"Try to get a resume on one page".
Actually, there is no rule for that. Of course, it's great to get a resume on one page but that's not the golden rule. When you try to push it all on a one-page resume, you start losing some detail and your resume becomes too generic and interchangeable. However, three pages are truly considered the maximum.
Another risk that you see is when people try to be complete in their resume by giving the same details to each job listed. Instead, try writing one line saying what you did in your past jobs.
Including a photo on your resume is also important. You want to connect with a person, and the interviewers want to see what they have in front instead of a piece of paper.
- Content
Make sure your resume is written for an experienced/ specialized hiring manager.
Never write your resume for a generic person. Write it for the experienced hiring manager (or your future boss), be specific. Don't try to explain the context, instead, include some undeniable details. When describing an experience, a project, or a research work, include details that only specialists understand. This will make you stand out from generic resumes.
For instance, if you have worked with a programming language, not just list it but also name what version you have used, and what libraries you have used most.
How to choose a domain or industry?
Try to be specific, generic choices on your resume will not make you stand out. Choosing an industry means that you have an intrinsic motivation to work for a certain industry or company.
Overcoming a few challenges
- Lack of work experience
The first lines on your resume stick in the hiring manager's mind. If you start saying you just graduated that's what they will remember "she is a graduate". Instead, you can start saying you just finished a project on a specific subject and that it greatly motivated you because now you know "xyz".
- Feel categorized according to the country you come from
Many people feel that this can happen but in fact, this is not true. You will not be categorized by the country you come from. Also, if you were being judged by your country you probably wouldn't want to work for that company.
- Not having a local work visa yet
In The Netherlands, the work visa needs to be requested by your (future) employer. There are a few workarounds for this problem. For instance, if you studied in The Netherlands and you are a non-European citizen you can have an orientation year to stay and find a job. There is also a program for the global top 200 universities (from any country), so if you studied at one of these universities you can apply in The Netherlands for the orientation work visa. There also is a list of the Dutch immigration service, called IND, and this list mentions all employers that are allowed to employ non-European knowledge migrants.
- Not hearing back after sending a CV
Unfortanelly this is very common problem. The only thing here is to be patient and continue applying for other companies.
- MOOCs on CV
There is no universal rule about MOOCs (Massive Open Online Courses), some people like it some don't. However, what you need to highlight is the university/degree that you did from your official studies.
Prepare for the Job Interview
Table of Content
- Doing the interview
- Rounding up a talk
- Doubt afterward
Doing the interview
Before jumping into your interview, make sure to be prepared by reading about the company, domain/ sector your applying for. It's important to show that you are inspired and motivated to do the job. Therefore, you need to understand what is happening in this industry. Lookup key topics in that industry and learn some domain terminology.
Another important tip to make a good impression is to create a connection with the interviewer. There is always an opportunity to have some personal talk going before you really start the interview, so you can take this to create that connection and relax a bit.
Also, even if the interviewer doesn't ask for technical details you must find a way to give some evidence of your knowledge and skills . You must create that moment yourself and go into some details during the conversation.
Rounding up a talk
It's also important to round up the talk by cracking a joke, again, we are back to the connection with the interviewer.
Some examples:
"Does your city have a decent football team?" (read: I'm ready to move to your city).
"How much does the housing cost?" (read: I'm ready to live there).
Also, always ask what comes next and send a very short thank you email on the same evening. Use this opportunity to demonstrate that you have enjoyed the interview.
Don't stuck in Doubts
Don't get stuck in bad feelings after an interview. If you feel it didn't go very well, then it probably didn't. Just keep going to the next one and try to improve what you think you didn't do well.
Selling Yourself and Negotiation
After a job offer, begins the negotiations. In The Netherlands, people seems to feel uncomfortable to start negotiations. In The Netherlands you get a base salary, holiday money (8% of your salary) and there is a 30% ruling (when you get a tax advantage).
The immigration services have defined benchmarks salary that people must earn in order to qualify for the knowledge migrant work visa. Of course, these benchmarks are known by the HR people, so they know they can't negotiate down to that benchmark. Therefore, you can take advantage of this information to make a realistic proposal.
You can check this article to see more about the salary expectations in Europe and in The Netherlands.
Data Science Case Presentation
This is a summary of a presentation by Damiaan Zwietering - Developer Advocate Machine Learning at IBM - given at the Data Science Career Summit 2020. You can find a full video of this presentation here.
Damiaan Zwietering is a Developer Advocate at IBM. Before his current position, he was a developer, consultant, architect and sales engineer in the area of data warehousing, business intelligence and advanced analytics. Currently, he works as a developer advocate for data science, specializing in the practical application of machine learning and artificial intelligence.
Table of Content
- Collecting data
- Jupyter notebook
- Exploring the data
- Data modeling
Collecting data
The first step in a data science project is to look for data, in this case presentation, we will be using COVID-19 data. One of the first organisations with available data on COVID-19 cases was the European Center for Disease Prevention and Control.
Jupyter notebook
Most of the data science is done using Jupyter notebooks. It's useful to set up an environment where one notebook tries to pick up its own data, work on something and produce an output file. Then, when we're finished we have a file that we can put into a production pipeline.
So to get started, we can create a notebook that downloads the file we want and starts doing some basic work. Here, we are using Pandas and NumPy packages to manipulate the data.
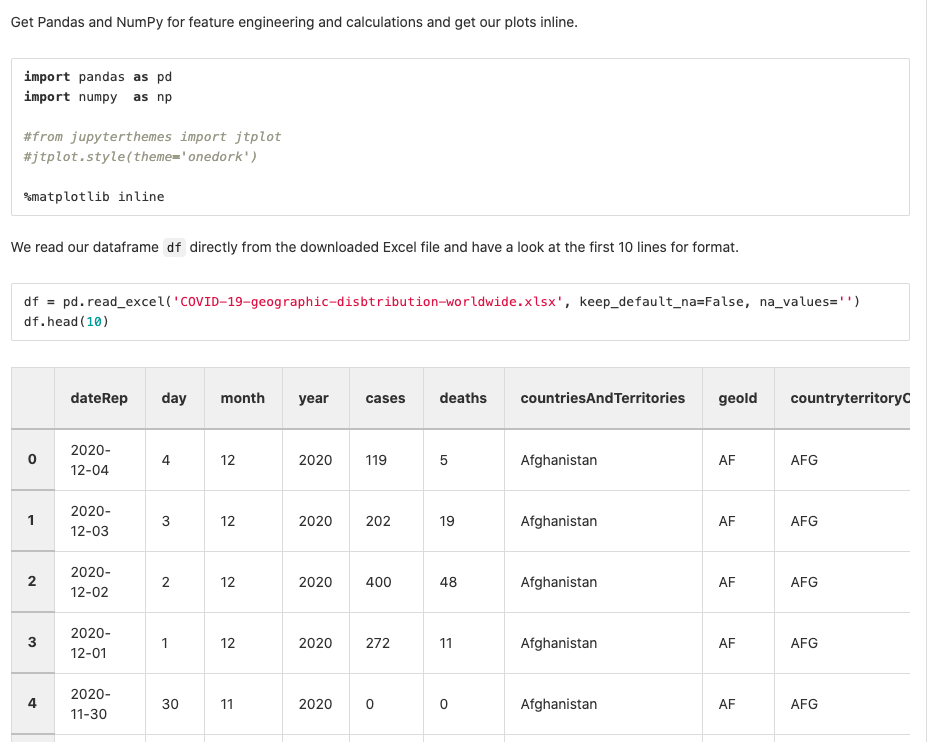
The next step is to have a first look at the data. This is a very important part where you have to check everything such as datatypes, extra spaces, duplicates, missing data, all kinds of things involved in the data cleaning process. When we have missing data we also have to check why the data is missing and decide how to deal with it.
If you want to be a data scientist you must like data cleaning because it's an important part of any data science project.
Exploring the data
Here, we picked three columns (cases, deaths, and geoId) and used a pivot table to facilitate the analysis.

Then, we can explore the data using data visualization. For instance, we can check the number of new cases per day for The Netherlands.

When looking at the graph for China (see below) we note some typical 'dirty data'. In February, they reported 15.000 cases but they didn't have a specific date set for those cases . That's why all cases were added to the same date. Then, when you draw the graph you get this huge spike.

In this kind of situations, we have to make a decision about this error. Communication is essential here. Talk to the people from where this data comes from and discuss with them how to handle it.
Also, before you start discussing where the error came from, always make sure that the problem is really in the data. Sometimes during the data manipulation, you might accidentally create errors, so always check everything. In this case, the error was indeed in the data, as we can see in the image below.
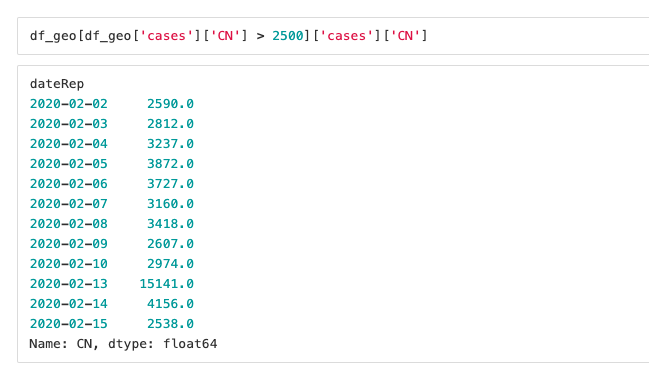
Before modeling, we have to understand what is the shape of an outbreak. For example, in the graph below we have two shapes, for The Netherlands and for Belgium. They have kind of a similar shape, there's a steep rise, then it plateaus and you also see that the tail of the distribution is a bit longer than the start.

Then, if we know what the general shape is and we have the rise of the shape, we might be able to predict the rest. We could do predictions about how many people would be infected or how hard the care system will be hit. The Gumbel distribution describes in a very general way the typical shape of outbreaks like this.
Data modeling
After exploring the data and making an assumption, we fitted the data to the first outbreak in The Netherlands. According to the Gumbel distribution, the model fits very well.

So now we have a description of our data, this is a time-series model. The first outbreak was at the beginning of the year and we can also see that there's a lot more data behind the curve. There's already a second shape curve effect and a big second wave. That's another big part of our investigation so we used an algorithm that tries to identify other Gumbel shapes in the data.
Below we see some kind of clustering based on this first model which tries to find out what are all the underlying outbreaks in this general curve that we have. The general curve is the blue one which is behind all the other curves.

We can see different waves, for instance, the first one is a smaller wave and it's a common wave seen in all countries at the beginning of the pandemic. The other waves happen specifically after holidays in The Netherlands. Thus, these are things that you would like to investigate along with a domain specialist or a behavior specialist, to understand why those waves happened at that time.